· 4 min read
Apple Intelligence, Private Cloud Compute
Edge-AI privacy innovation, security blueprint from Apple WWDC24 conference.
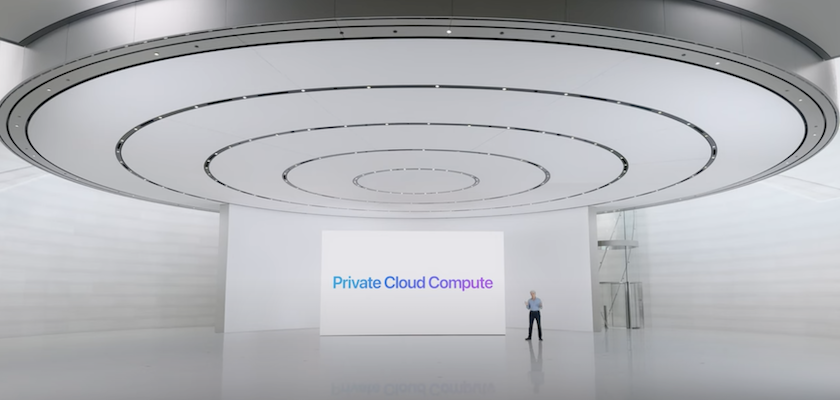
Apple Intelligence: On-Device Processing and the Future of Edge AI
Introduction
The latest developments from Apple WWDC24 conference have shed light on the company’s ambitious plans for Edge AI on-device processing. This innovation aims to revolutionize how AI is processed, particularly in scenarios where real-time decision-making is critical.
Apple WWDC24 Keynote : Apple Intelligence Reference
YouTube video at 1h14m43s
When you make a request, Apple Intelligence analysis whether it can be processed on device. If it needs greater computational capacity, it can draw on Private Cloud Compute, and send only the data that’s relevant to your task to be processed on Apple Silicon servers
Your data is never stored or made accessible to Apple. It’s used exclusively to fulfill your request. And just like your iPhone, independent experts can inspect the code that runs on the servers to verify this privacy promise.
In fact, Private Cloud Compute cryptographically ensures your iPhone, iPad, and Mac will refuse to talk to a server unless its software has been publicly logged for inspection.
This sets a brand new standard on Privacy and AI
Apple Intelligence: A Privacy-Preserving Approach
Apple has introduced its new intelligence system, which analyzes requests and determines whether they can be processed on-device or require the use of Private Cloud Compute for more complex tasks. This approach ensures that user data remains private and secure.
The company’s Private Cloud Compute feature enables users to send only relevant data to Apple Silicon servers for processing, without storing any sensitive information locally. This setup ensures that independent experts can inspect the code running on the servers to verify the privacy promise.
Embracing Edge AI On-Device Inferencing
The transition to Edge AI not only reduces operational expenses but also enhances real-time decision-making capabilities. Enterprises are increasingly recognizing the significance of this technology, particularly in light of the limited access to high-end GPUs like the NVIDIA H100, which often ties companies into public cloud ecosystems.
The Demand for AI-Embedded Software
Recent advancements in computing hardware have addressed some of the limitations associated with Edge NPUs. Apple’s M2 Pro and M3 chips have set new benchmarks in energy-efficient AI inferencing. Other powerful NPU edge computing solutions are emerging, including NVIDIA JetSon Orin, Qualcomm’s Snapdragon X Elite, AMD’s Ryzen AI, and Intel’s Gaudi3.
Real-Time Decisions in the Physical World
Edge AI has the potential to shine brightest in real-world, real-time decision-making scenarios. Autonomous vehicles, healthcare, and Apple’s Siri exemplify how AI can process device-specific information to make optimal decisions on behalf of users.
The Case for Distributed Tensor Mesh and Joint Inferencing
Standalone models often fall short in complex environments. The collective feed from multiple cameras or GPUs is necessary to inform autonomous driving decisions or obtain diagnostic confidence in medical devices. Distributed tensor mesh networks, where multiple models collaborate, can enhance decision-making accuracy and reliability.
Technology
Matrix multiplication is the dominant computation in Large Language Models (LLMs). Emerging techniques like tensor parallelism and distributed inference can alleviate scalability challenges. By dividing matrix multiplication among multiple devices, each device can perform a portion of the calculation, resulting in significant speedups.
However, synchronization between devices remains a major bottleneck due to communication links. Professional AI clusters often employ high-speed interconnects, but even these have limitations. Optimizing data requirements for synchronization can significantly reduce overhead.
Trust: User Privacy
User privacy is crucial for every enterprise. Apple’s announcement aligns with GEHC’s work in the MEDTECH world, emphasizing the importance of security engineering and privacy requirements.
Conclusion
Edge AI on-device inferencing represents a paradigm shift in enterprise strategy, promising enhanced real-time decision-making and cost efficiencies. By embracing advanced NPU technologies, harnessing distributed inferencing models, and overcoming traditional processor limitations, businesses can unlock unprecedented levels of agility, accuracy, and efficiency.
The strategic imperative is now for enterprises to integrate cutting-edge AI technologies into their core operations, driving innovation while safeguarding operational budgets. This will cement their competitive edge in an increasingly AI-driven world.
Credits : Unovie.AI