· EdgeAI · 3 min read
Vision AI for Logistics
Streamlining Processes and Empowering Decisions
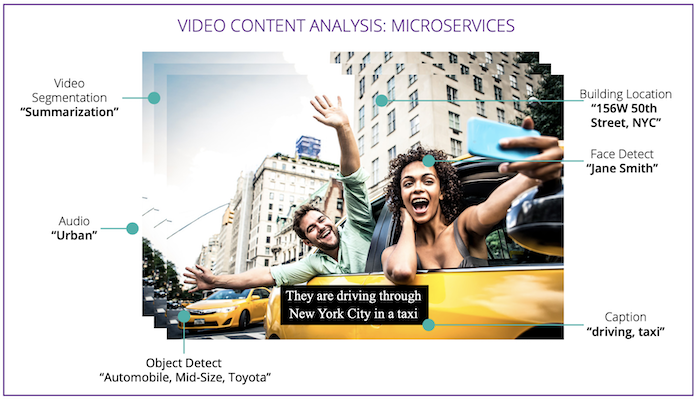
Overview
Vision AI has the potential to revolutionize logistics by automating complex processes, improving accuracy, and empowering data-driven decision-making. This article explores the transformative power of Vision GPT in streamlining logistics operations.
Challenges
- Limited availability of comprehensive visual annotations
- Absence of a unified pretraining framework with a singular neural network architecture seamlessly integrating spatial hierarchy and semantic granularity
- Existing datasets tailored for specialized applications heavily rely on human labeling, which limits the development of foundational models capable of capturing the intricacies of vision-related tasks
Vision Models
Phi-3-Vision-128K-instruct
Microsoft recently released Phi-3, a powerful language model, with a new Vision-Language variant called Phi-3-vision-128k-instruct. This 4B parameter model achieved impressive results on public benchmarks, even surpassing GPT-4V in some cases and outperforming Gemini 1.0 Pro V in all but MMMU.
FaceBook-Chmeleon
Chameleon 🦎 by Meta is a unique model that attempts to scale early fusion. Early fusion on the other hand attempts to fuse all features together (image patches and text) by using an image tokenizer and all tokens are projected into a shared space, which enables seamless generation.
Florence-2 Vision Architecture
Built by Microsoft, the Florence-2 model adopts a sequence-to-sequence architecture, integrating an image encoder and a multi-modality encoder-decoder. This design accommodates a spectrum of vision tasks without the need for task-specific architectural modifications, aligning with the ethos of the NLP community for versatile model development with a consistent underlying structure.
Key Capabilities
Inventory Management Recognition
Identifies items within images (e.g., pallets, crates, shipping containers) using convolutional neural networks (CNNs) and transformer models. This enables efficient tracking and tracing of inventory across supply chains.
Route Planning Understanding
Connects visual concepts with corresponding routing information, accurately interpreting descriptions of routes and transportation modes.
Supply Chain Contextual Reasoning
Analyzes the context surrounding shipments in images, discerning nuances often missed by traditional computer vision models, such as damage or tampering.
Transforming Logistics Processes
Vision GPT’s unique capabilities offer significant benefits across various logistics domains:
- Streamlined Warehouse Operations: Automates complex inventory management tasks, extracting crucial information like item locations and quantities.
- Enhanced Freight Audit Review: Analyzes shipping documents, matching line items with carrier details, rates, and insurance policies. Identifies discrepancies and potential issues, streamlining the payment process and reducing errors.
- Improved Route Optimization: Assists in analyzing logistics data, aiding dispatchers in finding the most efficient routes and supporting faster, more accurate delivery schedules.
Model Maturity
To ensure optimal performance:
- Utilize Vision GPT models specifically pre-trained on logistics-related datasets
- Prioritize deploying models locally and pretrain them with customer-specific data to ensure complete control over features, versions, and expected behavior
- Have strong Pen-Testing Capabilities built in to ensure these models cannot be compromised by hidden OCR messages that are not visible to human, but will affect the automated decisions upstream.
Conclusion
Vision GPT holds immense promise for transforming logistics operations by automating complex tasks, improving accuracy, and empowering data-driven decision-making. By addressing concerns and implementing best practices, logistics organizations can leverage this powerful technology to enhance operational efficiency, improve accuracy, and ultimately achieve better outcomes.
Credits : unovie.ai